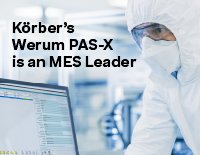
In this article, we delve into a Bioprocess Design of Experiments or “DoE”, a core technology for the organization of bioprocess development. Arguably, DoE is one of the most valuable tools in process development and manufacturing problem-solving. DoE has shaped the way we think as scientists and engineers about doing experiments. As scientists in bioprocess development, we always have limited resources to investigate, explore or optimize bioprocesses. DoE is one of our primary tools to use our resources most efficiently. DoE brings structure and efficiency to process development. This saves companies a lot of money. And, interestingly, DoE also brought great organizational change to organizations that adopted this technology.
Read here about how biotech organizations adopt DoE in various stages of the bioprocess lifecycle. Read about how DoE triggers organizational change and a re-thinking of our bioprocess development practices. But adopting DoEs is not always straightforward: Be aware of common pitfalls and follow DoE best practices for bioprocess development.
Comments
No comments