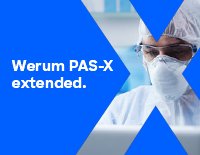
Many companies face the challenge of reducing the developmental timelines and efficiently optimize the process thereby bringing the drug to market in a faster way. In this article, we introduce a dynamic modeling approach that contributes to a new era of rapid and efficient bioprocess product development.
In a world of the ever-changing landscape of bioprocess development, the importance of faster process development while keeping product quality and process efficiency intact is the need of the hour. This not only helps the manufacturers to improve their cost of goods sold (COGS) but also makes the drugs available to patients at a faster rate which brings both parties in a win-win situation.
The increasing demand for life-saving medications to the market has led to the adoption of state-of-the-art tools and techniques. A solution to tackle this complex situation is to make use of innovative methodologies. In this article, we will demonstrate one of these methodologies, dynamic modelling, as an enabler to reduce experiments and hence fasten process development.