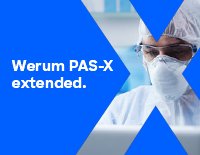
Everywhere you turn, someone talks about data analytics. Biotech companies, CMOs, big-pharma seek ways to gain competitive advantages through data analytics. Furthermore, regulators call for the use of advanced data analytics and data integrity for the entire bioprocess lifecycle. However, applying the right data analytics in bioprocess development and GMP manufacturing is challenging and very different from business analytics or statistics in clinical trials. High analytical method errors. A low number of runs/observations. How to deal with that?
Bayesian bioprocess data analytics offers a solution that shall not be overlooked. Bayesian is a different way to think about and model data. This article delves into the basics of this exciting technology and explores applications in bioprocess development and manufacturing.
Kommentare
Keine Kommentare